
Bringing data science learning opportunities to all students requires action from all of us. When we work together as a coalition, we can raise awareness, generate demand, and move the needle.

Calls to Action:
Schools & Districts
Many schools roll out data science as part of their math curriculum, others infuse data science concepts across disciplines. Learn about K-12 implementation models here.
Your calls to action:
-
Offer a data science course at your school
-
Update graduation requirements to accept data science as a mathematics or CTE course
-
Train teachers across disciplines to incorporate data literacy into their lessons
-
Host a town hall or start an exploratory committee with school and parent stakeholders to start talking about data science and create a district plan
Who's taking action:
.png)
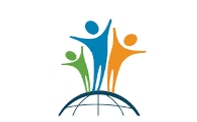
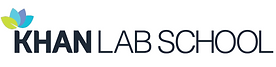
San Diego is rolling out data science to 120k students across TK-12 mathematics learning with the CourseKata curriculum.
Frederick County Public Schools is implementing the Virginia Data Science Standards of Learning through a standalone high school course.
Khan Lab School is piloting a new data science course using the Merge & Purge model to build data science learning into the math sequence and expand access to students. Learn more.
Colleges & Universities
You’re setting the standard for what quality higher education looks like, producing innovative research, and signaling to K-12 educators how to prepare students to access higher education.
Your calls to action:
-
Update admissions requirements to accept data science in fulfillment of a math requirement
-
Offer a data science course in fulfillment of a general education requirement
-
Emphasize data literacy in pre-service teacher training
-
Partner with a local district to offer data science professional learning opportunities to teachers
Who’s taking action:
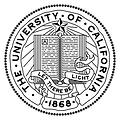
The University of California and Cal State systems accept data science for university admissions. Learn more.
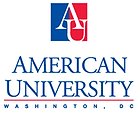
American University is partnered with DC Public Schools to provide free master’s level data science course work to high school teachers in the district. Learn more.
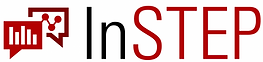
State & Federal Policymakers
You’re determining what student and teacher success looks like across the country while driving research and innovation in education.
Your calls to action:
-
Build data science into state standards and frameworks
-
Develop new programs for student learning and teacher professional development in data science
-
Prioritize funding for data science education and research
-
Introduce legislation prioritizing data science
Who’s taking action:

Arkansas Department of Education is investing $1.5M in computer science teacher training, including for advanced data science standards.

10 US Senators are advocating for expanding access to data science education. Learn more.
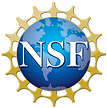.png)
ED, NSF, IES have prioritized data science education in recent grant programs. Learn more.
Organizations & Industry
You're providing the resources, programming, and support to expand high-quality learning opportunities.
Your calls to action:
-
Develop high-quality data science content and programming.
-
Partner with schools, districts, and state education departments to put your resources into the hands of teachers and students, help expand data science learning opportunities.
-
Offer services or mentorship to students and teachers.
-
Talk about what you’re doing to raise awareness of the importance of data literacy.
Who’s taking action:
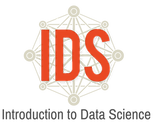
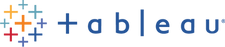.png)
Introduction to Data Science is the first full-year high school data science course and is being taught in more than 65 schools nationwide. Learn more.
Tableau is offering its software, learning resources, and curricula for free for teachers and students. Learn more.
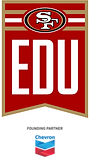_PNG.png)
SAP & the San Francisco 49ers are partnered to develop a virtual learning series and full course curriculum demonstrating the role of data science in football. Learn more.
Teachers
You are the most crucial link to introducing data science into classrooms! You are delivering instruction to your students and engaging them in powerful learning opportunities.
Your calls to action:
-
Incorporate data science learning modules or activities into your lessons.
-
Get your student working with data in their class assignments.
-
Talk to your students about the importance of data literacy.
Learn about resources you can use in your classroom here.
Individuals
You are powering interest, demand, and change at the grassroots level.
Your calls to action:
-
Demand data science learning opportunities from decision-makers in your school, university, or local communities.
-
Talk to your kids about the importance of data literacy.
-
Grow your own skills in data literacy.
Visit our resource center to learn more about data literacy and expand your data science knowledge.
